Big Data, Better Cancer Care
by Rebecca Dzombak
By keeping people at the center of data and machine learning efforts, Rogel researchers are creating more personalized and equitable patient care.
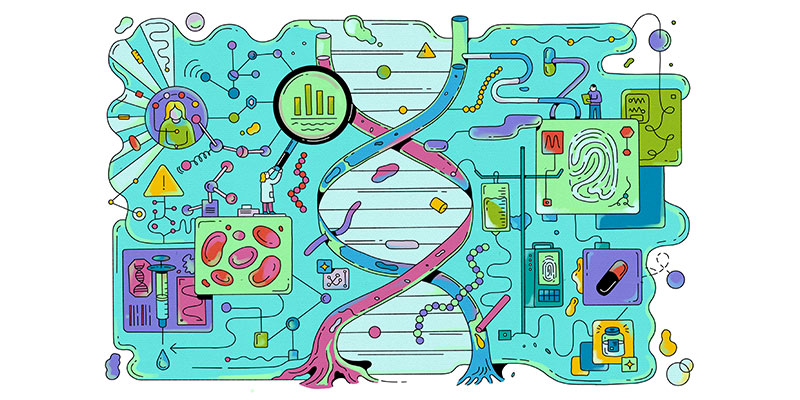
Illustration: Kathleen Fu
The human touch of the care team plays a central role in every patient’s experience, from bedside manner to scribbled notes. But the use of artificial intelligence and machine learning is becoming more common in health care, improving patient care in previously unimaginable ways.
Researchers at the Rogel Cancer Center are helping to hone these evolving tools, setting standards for patient data collection, storage and analysis. By putting patients first in health data science, Rogel researchers are creating treatments that can be more personalized, using technology to detect adverse reactions sooner, and constructing large digital databases of cancer patients to deliver more equitable care, all while keeping health care a human experience.
Building Data We Can Use
For a dataset to help patients, it must be good: useable, high-quality data, with biases identified and enough samples to be statistically robust. That may seem basic, but until now, there haven’t been standardized ways of recording patient and treatment information.
The lack of standardization is also a critical barrier to building large, representative datasets in the first place, limiting how agencies administering clinical trials can combine and use the resulting data.
Several Rogel researchers have dedicated recent years to developing nomenclature standards for U-M and their collaborators. The first step, deceptively simple, is getting everyone to document the same information in the same way.
For Charles Mayo, Ph.D., a medical physicist and data scientist who joined Rogel in 2015, an interest in health data science was sparked by his research into radiation therapy dosing that explored why toxicity levels vary across individuals and tumor locations.
"We never want the cure to be worse than the disease," Mayo explains. "As we treat each patient, we want to be able to take what we’ve learned from that experience and help improve treatment for the next patient. Learning from high-volume, comprehensive, real-world data could help generate new evidence-based insights and hypotheses."
But as Mayo searched the literature for case studies of radiation toxicity, he found only dozens of patients, not the thousands he expected. Because the data weren’t standardized and digitized, it was nearly impossible to collect the information he needed.
"This data was gathered manually, as it still is today," he says. "You’d have to comb through people’s charts to find that information, and it’s challenging because people are inexact in their language. We lack any sort of standardization or nomenclature to ensure we’re all documenting the same things in the same ways."
Even if the necessary data exist, it’s impossible for a computer program to automatically compile and use the data without standardized ways of recording information like a tumor’s location or patient demographics. Even with increasingly sophisticated technology, nothing matches up.
"It was just naming chaos," says Elizabeth Covington, Ph.D., a medical physicist at Michigan Medicine who worked with Mayo on the project.
To finally standardize radiation oncology nomenclatures, Mayo led an international task group in the American Association of Physicists in Medicine. They set about establishing a standardized nomenclature for everything from disease staging to seemingly obvious things, like how to refer to a body part.
"Humans are endlessly creative making up names, but we tend to be inconsistent over time," Mayo said. "For example, when we looked at records from historic plans, there were hundreds of character strings each pointing to ‘right optic nerve."
The resulting schema, called TG-263, was published in 2018 and quickly implemented in clinical trials. (Their choice for right optic nerve: "OpticNrv R"). Standardized naming enabled automated data compilation across trials and expanded the range of questions the datasets could answer. Covington is now leading the first update of TG-263, including French and Spanish translations and expanding overall coverage. Covington and Mayo hope international standards will facilitate international collaboration and larger, more diverse patient databases.
Agreeing on how to name concepts was only part of the problem; patient data still needed to be efficiently and accurately collected for researchers and clinicians to benefit. So, for Michigan Medicine, Mayo spent six years building a database called the Michigan Radiation Oncology Analytics Resource (MROAR). It compiles standardized, comprehensive data from every patient they treat, from demographics and background to lab results, medicines and chemotherapy. MROAR enables researchers to pull large swaths of privatized patient data to explore treatment options and helps clinicians see all their patient’s data at a glance, rather than navigating through multiple records.
AI and machine learning have great potential, but the technology wasn’t the hardest part of that process, Mayo says. It was the people.
"The temptation is to think it’s all about the technology," he says. "It turns out, it’s mostly about practice—whether people have and are using tools to enter data consistently, in a way that allows automated, electronic extraction. Getting to that point can take a lot of persuasion."
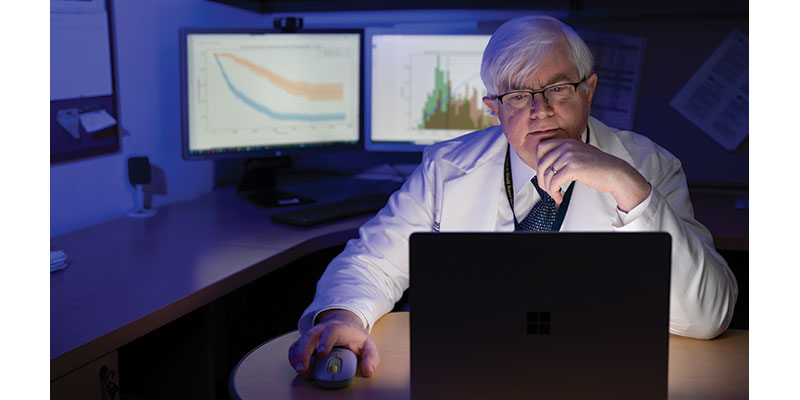
Charles Mayo, Ph.D.
Photo credit: Erica Bass
Avoiding Biased Data, Saving Lives
Standardized documentation isn’t the only quality issue with building datasets. Identifying biases, and avoiding them when possible, is critical for health data scientists. Failing to do so could result in subpar, or even harmful, treatment.
"Introducing or propagating bias when we’re training models is always a concern," Covington says. "We have to be thoughtful about the data. For example, many clinical trials historically over-represented white patients. But trials may not have recorded the patient population demographics, or done so inconsistently. We really have to know what’s in the data before using it to inform patient treatment," she says.
Daniel Hertz, Pharm.D., Ph.D., pharmacogeneticist at Rogel, agrees that bias in datasets can be problematic.
"There have actually been cases where clinical trials have worsened outcomes for non-Europeans because the cohorts the algorithms were trained on were only Europeans," Hertz says. "We need larger and more diverse cohorts so that we can actually use the results to help all patients."
Even as researchers are more aware of demographic biases in clinical trials and implications for treatment recommendations, the health equity gap will be slow to shrink if demographic data continues to be collected sporadically across institutions.
Relevant population information beyond the "basics" of gender, age and race is even less likely to be collected, Covington says. Other factors that can impact health, such as socio-economics, disability and caretaker status, could provide useful information to risk factors, but these are not commonly collected.
Even a person’s address can be indicative of risk factors: If a community has fewer resources, an individual might receive a standard screening later than ideal, causing them to come to U-M for treatment with a later-stage cancer, Covington says.
"If we’re not monitoring all of these factors and collecting that data, then we don’t know if people are getting equitable care," she says. "We can’t learn anything from the data unless we’re asking the right questions.
Tailored Treatment
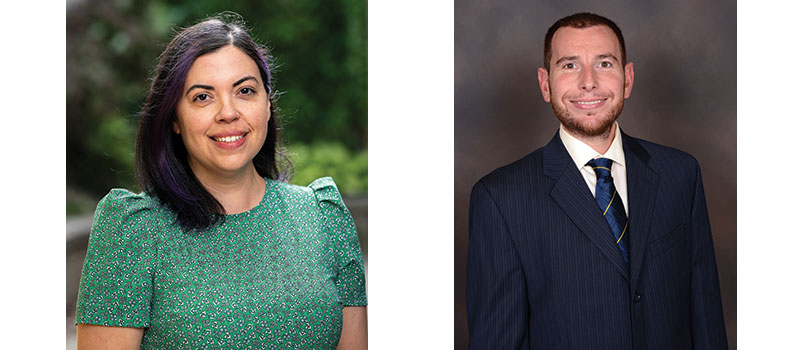
Elizabeth Covington, Ph.D. (left) and Daniel Hertz, Ph.D.
Photo credit: Michigan Medicine
Hertz has been working on making treatment more personalized for over 15 years. He uses algorithms trained on large datasets of genetic data and chemotherapy dose levels to predict individualized toxicity levels, with a goal of decreasing the risk of a severe reaction to treatment.
Wearing your heart(rate) on your sleeve
Wearable health technology, such as heart rate sensors, can enable real-time health data collection in an accessible and equitable way. Sung Won Choi, M.D., is a pediatric oncologist at Rogel who specializes in bone marrow transplant. One of the main adverse outcomes of transplant is graft versus host disease, or GVHD. The diagnosis often involves invasive biopsies.
Early in her career, Choi was interested in identifying blood-based biomarkers of GVHD; over time, that interest spread to other tools that could provide signs to predict and monitor adverse reactions to treatments.
"If we can identify markers of complications before they happen, particularly through non-invasive methods, we could ensure patients receive timely care," Choi says.
Fever is an early hallmark of GVHD, as well as for a cytokine response to CAR T-cell therapy, a treatment where a patient’s own T-cells are modified to better recognize and attack cancer cells. Choi and collaborator Muneesh Tewari, M.D., Ph.D., professor of internal medicine and biomedical engineering at Michigan Medicine, thought wearable tech could help.
They got their chance to explore the tech as COVID-19 hit U-M’s campus. Their study of heart rate in U-M college students with COVID-19, published in Cell Reports Medicine, revealed that FitBit-detected heart rate changes correlated with symptoms in a predictive manner. Wearable, commercial-grade tech could work.
Choi and Tewari were also exploring the use of wearable tech to detect fever in cancer patients who had received CAR T-cell therapy. The results, published in Cancer Cell, were promising. Even a relatively low-tech wearable temperature sensor could alert the onset of fever before a normal thermometer.
The approach isn’t ready for "prime time," Choi says, but it shows promise. In one case, a patient had a detected fever and called the hospital, who had them come into the emergency department.
"Because of that, they received antibiotics sooner than they would have otherwise and possibly prevented a major case of sepsis," Choi says. "We have a few anecdotal cases, but they illustrate how early markers of complications can lead to timely interventions."
Because they’re using commercial-grade wearables, more people could have access to this type of care, Choi explains. "It wouldn’t make up for having high-quality, local health care, but it could help narrow that gap and improve care for people who, for a number of reasons, might not seek care otherwise."
It’s also cheaper, she adds. "What good is a million dollar device if patients won’t wear it?" And because many people are used to wearable tech monitoring their health, as with steps and heart rate, they might be more comfortable sharing those data for research.
"But it’s still very personal," Choi says. "It’s the stamp of your heart."
A primary focus is toxic responses to fluoropyrimidine-based chemotherapies, which are commonly prescribed for solid tumors of the head, neck, breast and gastrointestinal tract. Up to 30% of patients experience severe toxicity in response to these drugs and up to 1% will die as a result. Predicting who is likely to have this toxic response and reducing dose levels could cut down those risks.
"It just so happens that for fluoropyrimidines, there are some very strong genetic predictors that would allow us to test and identify appropriate dosing for patients," Hertz says.
In this case, the predictive gene is for DPYD, the primary enzyme active in fluoropyrimidine metabolism. Hertz and his colleagues discovered this using a database of 582 patients from the Michigan Genomics Initiative to test whether a DPYD variant predicted fluoropyrimidine toxicity. They found patients with DPYD variants were two to four times more likely to experience toxicity.
Those results, published in Pharmacogenomics in 2021, cleared the way for exploring DPYD and toxicity in other treatments, as well as using genetic testing prior to treatment to detect DPYD and adjust dosing accordingly. Hertz and colleagues set up an alert system for patients in the Michigan Genomics Initiative database who were positive for DPYD. When these patients were referred to an oncologist, an alert went out to the care team. A case study, published in Clinical Pharmacology & Therapeutics in 2023, documented one early success with this program.
"Within the first 24 hours of activating that alert system, we got an alert that someone who had previously tested positive for DPYD was scheduled to receive fluoropyrimidine chemotherapy," Hertz says. "We called the patient and explained that based on their genetic information, they were at high risk of severe toxicity, so we were going to work with their medical oncologist to alter their treatment plan. They were extremely excited and grateful that we had detected this, and that we could give them safer treatment."
"It was probably the most rewarding moment of my career," Hertz says.
The potential for using large genetic databases to improve treatment is real and immediate, as Hertz’s work has demonstrated. Recent technological advancements make this work possible.
"We’ve seen dramatic improvements in the availability of data, efforts to build large-scale databases and the analytical approaches," Hertz says. "AI, machine learning and other statistical approaches allow us to process all this data and find potentially lifesaving patterns."
But data sharing across borders, and even between institutions, remains a barrier to building databases large enough to improve treatments for the rarest cancers using this approach. Out of a sample of 100,000 patients, a few thousand may have cancer, and a hundred or so may have received a particular medication. That limits what researchers like Hertz can do, statistically.
For some researchers, the statistical limitations of small datasets are worth it because they might be very high quality, whereas larger datasets are "messier"—the data need to be filtered out and cleaned before researchers can use them. Mayo rejects this as a false choice. "It’s not ‘either/or,’" he says. “We need to use both."
While successes like the DPYD alert and other predictors of toxicity have piqued people’s interest in participating in data sharing—doctor and patient alike—there’s still reluctance to change workflows and treatments based on algorithms.
"We’re in the very early days of some of these models," Hertz says. "There’s naturally some skepticism of any new strategy, and there’s some hesitancy among clinicians to use algorithms and models. But as clinicians start to see the power and possibility in electric health records and better understand where these recommendations are coming from, trust will grow."
Mayo says that some degree of skepticism in physicians can be important. "There are so many factors they’re considering when deciding on a treatment plan. A patient is sitting in front of you, and you want to be confident when you tell them what the best course of action is." Demonstrating the reliability of algorithm-based information will be crucial for building that trust and enabling more predictive patient care to occur.
A Data-Driven, Patient-First Future
A broad implementation of algorithms to predict severe reactions and inform patient treatments may be in its infancy, but datasets and algorithms can inform hypothesis generation and clinical trial design now. Researchers can use machine learning on large datasets to find the most significant factors tied to outcomes, test those associations with other models, and build clinical trials around those factors.
"Instead of looking at dozens of candidate features, we can test hundreds and use our algorithms to narrow focus to the few that emerged as being relevant during data processing," Mayo says.
Hertz’s case study of a genetics-based alert potentially saving a patient’s life drives home an ethos central to these researchers’ perspectives on the role of data and data-based care: Each patient is far more than one point on a graph. Every piece of data is personal and valuable, and Rogel physicians and researchers feel privileged when patients trust them to use their data to advance care for others.
Ultimately, as data standards improve, datasets grow and machine learning tools evolve, more patients will be able to benefit from data-informed personalized treatments. But the people on patients’ care teams won’t be replaced by machines any time soon.
Continue reading the 2025 issue of Illuminate.